Three scholars affiliated with Johns Hopkins have evaluated cryptocurrency investing, in a new paper available at SSRN, and have concluded that “near-term cryptocurrency markets are semi-strong form efficient.” That bit of finance theorist jargon means that all publicly available information gets discounted quickly into an asset’s price, so that neither fundamental nor technical analysis can reliably produce alpha. In an ideal, or strong form efficient market, even private or “insider” information gets discounted into an asset’s price, so that insider trading (even aside from the question of discovery and punishment) won’t produce alpha either. The Johns Hopkins scholars, Jim Kyung-Soo Liew et al, don’t make the strong claim, but they do make the semi-strong claim for cryptos, and that still represents a remarkable fact about those markets if their claim holds up in the face of continuing research. The research that reached this result involved rolling prediction analysis run on 57 cryptocurrencies with 11 artificial-intelligence algorithms. Although some information sets, employed by some families of algorithms, did better than others, the general result was that “predictability may be difficult,” and day traders hoping to make a quick buck face grave challenges. Literature Review The paper includes a literature review. One 2014 study, published by Isaac Madan and associates at the University of Stanford, used three machine-learning algorithms to try to predict bitcoin prices. They confined themselves to predicting the sign of the coming day’s price move (that is, whether it would be up or down). With this binary focus, their algorithms were very accurate, at best 98.7% accurate. The following year another group of Stanford researchers sought to predict the hourly change, up or down. Their accuracy rate was considerably less impressive: only 55%. That’s a huge difference: near perfection on the day-to-day predictions, neat coin-flip results on the hour-to-hour predictions. Yet another study, by Jermain Kaminsky, of the Massachusetts Institute of Technology, tested the hypothesis that one could successfully predict bitcoin movements from a database consisting of twitter posts, but came up with the disappointing result that twitter references to bitcoin merely mirror, they do not predict, the actual market in bitcoins. The Kaminsky result is of course perfectly consistent (although it does not require) the inference that the bitcoin market is efficient, whether in the strong or semi-strong sense of the term. Since the faraway day of those studies (three to four years ago!), the scholarly world has dropped the assumption that “bitcoin” is the paradigmatic or central cryptocurrency. As the Johns Hopkins authors observe, there are as of last count 1,524 cryptocurrencies, traded on many different exchanges. These authors narrowed their own database down to the top 100 of them, as defined by market capitalization as of February 18, 2018, and excluding from consideration those with a “relatively short history.” Volatility The “top tree,” bitcoin, ripple, and ether, have fairly low standard deviations. Looking to daily price returns on bitcoin in the period from January 2015 to February 2018, the standard deviation is 0.0403. For weekly price returns, the SD is 0.1053. For ripple, the same period, the corresponding two numbers are: 0.0914 and 0.2808. For ether, the SD numbers are: 0.0798 and 0.2514. Those are the established, low-vol cryptos. But given the presence of lots of other less ‘respectable,’ less according-to-Hoyle cryptos, the average standard deviation for the whole of the top 100 is a good deal higher. The number for daily SD for the top 100 is 0.4701. For weekly SD, its 0.9940. Correlations and Arbitrage The Johns Hopkins authors also look at correlations. They find that bitcoin is “barely correlated to any traditional assets at the daily level[and] exhibits a slightly positive correlation to S&P 500, MSCI, USD, Gold, and Commo, while demonstrating a negative correlation to [emerging markets] and VIX.” In addition to his position as assistant professor of finance at Johns Hopkins Carey Business School, Dr. Liew is the CEO and founder of SoKat Consulting LLC. His co-authors are: Richard Ziyuan Li, a recent graduate of the Johns Hopkins financial mathematics masters program; and Tamas Budavari, an assistant professor in the Johns Hopkins Whiting School of Engineering Department of Applied Mathematics & Statistics. They observe that the prices quoted on various exchanges can vary widely. That would seem to be exploitable for returns, except that it has been very difficult to take a short position on cryptos, at least until December of last year when the CBOE and CME introduced bitcoin.

←
Back to Portfolio for the Future™
The Efficiency of the Markets in Crypto-Currencies
June 28, 2018
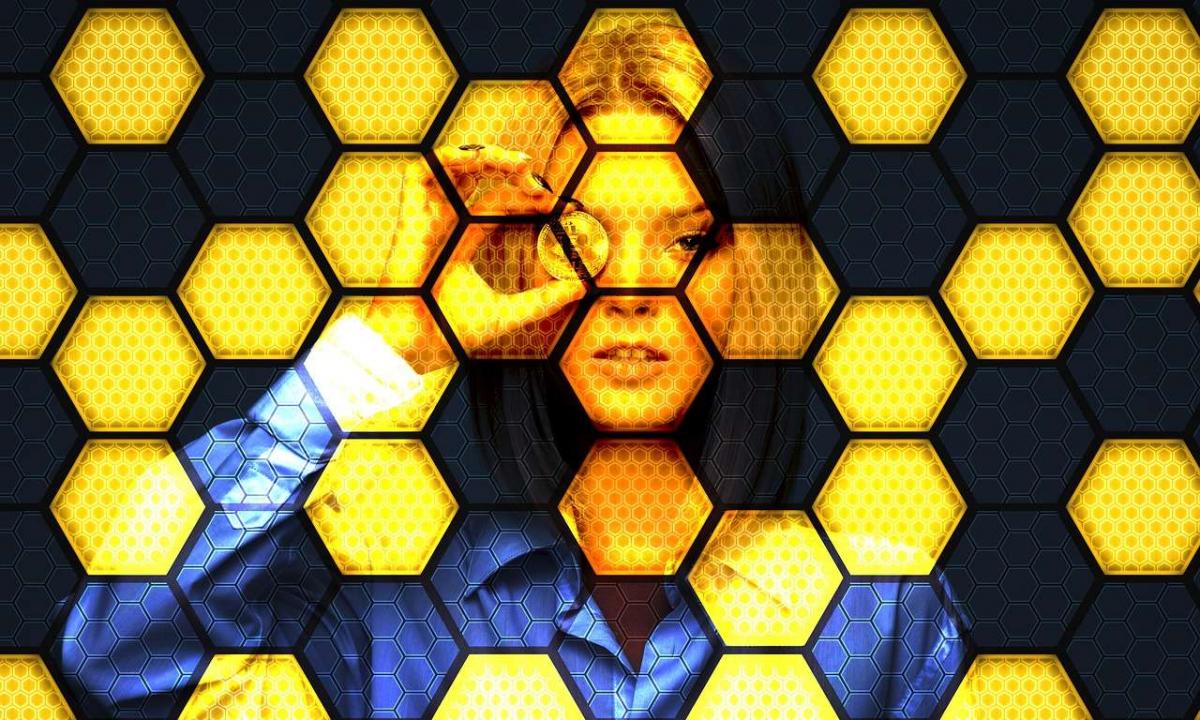