A new paper by J.B. Heaton, forthcoming in the Journal of Financial Transformation, offers a skeptical view of the algorithmic trading of securities, its actuality and its potential. Heaton is a lawyer (admitted to the bars of both Illinois and New York) and has a Ph.D. in finance, University of Chicago. In the forthcoming paper he contends that with only “a tiny handful of exceptions” (read “Renaissance”) such trading doesn’t produce exceptional results. The problem goes far beyond what one addresses by saying that the field is new and still developing, that machine learning will get better, that Big Data will get even bigger, and so forth. Heaton asks us to contemplate Citadel LLC. This huge hedge fund “returned only about 13 percent in 2017,” which was short of the S&P 500 gain. Yet the S&P gain would have been “available quite inexpensively to anyone with the money to open an account at Vanguard Group.” Does Citadel compensate for underperformance in bull markets by preserving capital in bear markets? Heaton says that it does not, “Citadel fell nearly 60 percent in 2008, far more than the S&P 500 index.” Or if one’s memory is taxed by the need to think back nearly a decade, consider early February of this year, when turmoil hit the U.S. stock markets for reasons still not entirely clear. Did a heavily quantitative, algorithmic approach to investing keep one out of trouble at that moment? No: Winton, AHL, and a large quant fund under the wing of Lynx Asset Management all took big hits at that time. Finding the Dog in the Photo In order to understand the “whys” of this generally unimpressive performance by the quants, Heaton says it is necessary to take a look at what machine learning (and a subset thereof he calls “deep learning”) is and entails. Machine learning is “incredibly powerful with data patterns that are stable.” It is possible to teach computers to decide whether there is a dog within a photograph. This is complicated, not merely because the word “dog” describes a lot of different beasts, but because in a particular photo the dog present may be partially hidden, or visible from an odd angle, etc. Still, the complexity of the task is not the problem. Machine learning can start with a rough model of what a photo looks like when there is a dog in it, then train the model over time, testing on new data or on data that had been set aside to help that first rough model get its start. It will get good at the task over time, and that will happen because dogs are what they are and will stay what they are. Financial data, on the other hand, doesn’t have the stability that the algorithms work from and rely upon. Part of the reason they don’t is that efforts to work from and with them become predictable and create arbitrage opportunities. As Heaton puts it, “Quants are all trying to hire the same kinds of people, educated at the same institutions, and trained in the same methodologies. As a result, quant trading is largely … a commodity business and competition to exploit signals looks a lot like competition to buy and sell identically-graded wheat.” So the dog doesn’t stay a dog, and sometimes evolves quickly into a new species while the investor is still taking a position on the dog. But even if the dog in the photographs stays a dog, the odds are good that it isn’t worth the money that the investor is paying for the computer power. Marketing Beta “Indeed,” Heaton says, “the marketing genius of firms like Dimensional Fund Advisors and AQR is that they implemented simple academic factor model-based investing as if it offered superior risk-adjusted performance when that superior performance is probably just compensation for economic risk that occasionally bites the investors who bear it.” In other words, Heaton seems to be saying, they market their ability to glean smart beta as if it is alpha. Heaton is making a familiar point, though from a somewhat novel point of view, informed by the language of computer science mixed a bit with that of finance. As his paper’s abstract says, investors “will continue to remain best-served by inexpensive and passive index products that themselves will be augmented by machine learning techniques to drive costs even lower.”
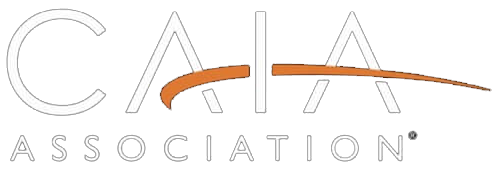
- Programs
- Events and Webcasts
- Resources
- About
- Official Merchandise

