By Keith Black, PhD, CFA, CAIA, FDP, Managing Director, CAIA Association The FDP Institute recently spoke with Al Yazdani, the chief data scientist and founder of Calcolo Analytics, regarding his forthcoming article in the Journal of Financial Data Science: “Machine Learning Prediction of Recessions: An Imbalanced Classification Approach.” The author will post the code used for this project so other researchers can replicate the results. The traditional method for predicting recessions is to use a Probit model, which is a generalized linear model that uses constant weights for predictors across all recessions. As a linear model, Probit is much less flexible than machine learning approaches which can capture nonlinear and interactive relationships between variables. The National Bureau of Economic Research (NBER) determines the dates that recessions start and end, but do so with a lag, as economic statistics such as GDP growth are not reported in real time. According to NBER estimates, the US economy spent about 14% of months since 1959 in nine declared recessions. The imbalanced classification approach is used, as the US economy is growing in about six times the number of months where it is shrinking. Members and candidates for the Financial Data Professional (FDP) designation are well aware of the models and metrics employed by Dr. Yazdani. Machine-learning models including support vector machines, random forests, and neural networks are employed with performance benchmarked to the standard Probit model. Metrics include precision, recall, accuracy rate, specificity, and area under the curve, among others. An ensemble model using random forests reported the best predictions. While Probit had an out-of-sample accuracy of 91%, precision of 59%, and sensitivity of 91%, the ensemble random forest model outperformed with statistics of 94%, 69% and 94%, respectively. Researchers should be familiar with their data, including understanding the frequency of the data available and the frequency of the variable to be predicted. All data should be examined and pre-processed to understand the cleanliness and form of the data, such as whether relationships appear to be linear or nonlinear. The longer the prediction horizon, in general, the less useful daily data may be, as daily data may be too volatile to predict long-run returns to stocks and markets. Predicting recessions, then, focuses more on monthly and long-term data, such as stock market returns, payrolls and unemployment statistics, industrial production, and interest rate data, such as 10 year Treasury yields, the Fed Funds rate, and the shape of the yield curve. It is important to note that all seven factors in Yazdani’s model were each previously reported in the academic literature as being predictive of recessions. Rather than downloading reams of data and asking the models to train themselves, this problem was built using the standard and proven intuitions of academic economists. Here is the schedule for future FDP webinars, along with the archived webinar and slides for Dr. Yazdani’s presentation. Learn more about the FDP exam and curriculum Interested in contributing to Portfolio for the Future? Drop us a line at content@caia.org

←
Back to Portfolio for the Future™
Seeking to Predict Recessions? Focus on the Forest—Not the Trees
July 21, 2020
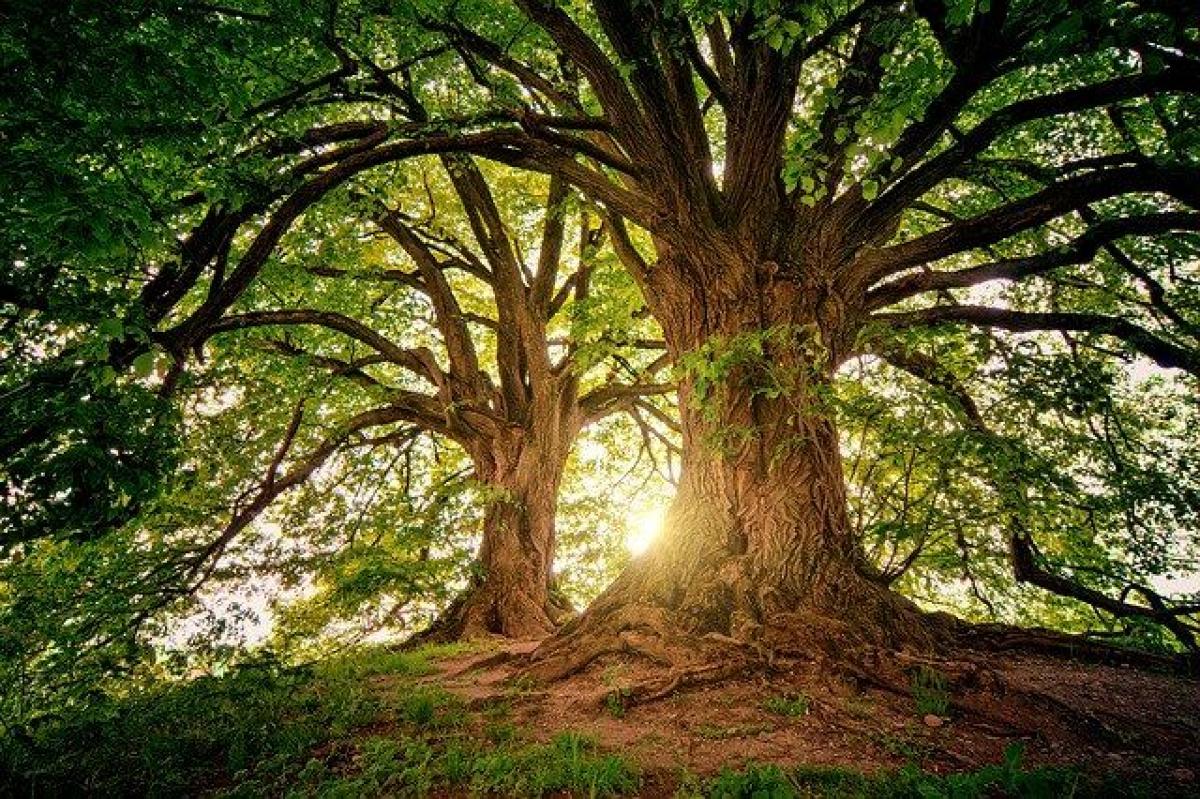