By Dan diBartolomeo, President and founder of Northfield Information Services, Inc. Based in Boston since 1986, Northfield develops quantitative models of financial markets.
Many investment professionals who use risk models make a common mistake. They assume that a risk model is working well if the amount of volatility realized by a particular asset or portfolio is consistent with what the model had predicted. They believe that volatility forecasts should be an unbiased estimator of subsequent realized volatility. This article will provide five different rationales as to why seemingly unbiased estimates of volatility are undesirable as risk measures, both statistically and economically. The implications of these arguments are that professional investors routinely take too much risk, back-tests and simulations fail to capture the true risk of strategies, and that evaluation of investment performance is biased toward perceiving luck as skill -- leading to upward biased performance related compensation. Even other risk vendors have been known to advocate for unbiased test results (bias ratio = 1). In essence, these providers are intentionally selling a model of volatility rather than a model of risk.
In more than thirty years of Northfield operation creating and selling analytical models of investment risk, we have had dozens (if not hundreds) of prospective clients attempt some sort of “back-test” or simulation to determine if our models were more or less effective than competing models. Not even in a single case was it true that these tests produced statistically significant results. In every case, we were able to point out flaws in how the experiments were designed, the size and independence of samples, and the evaluation of outcomes. Many of the tests simply ignored the concept that risk had to be evaluated over a defined future time horizon. Essentially, the tests were a coin flip. Most of the time the model that produced the lowest risk estimate was judged best. As an asset manager it is often nice to assert to your clients that you have low risk even if it is not true.
We first have to understand the pitfalls of thinking of volatility as a measure of risk. As a concept, risk relates to the potential for undesirable outcomes in the future and the consequences of those outcomes. Being in the future, risk cannot be measured. It can only be estimated. Volatility of investment returns can be measured in the past or estimated for the future. Even when estimated, future volatility of return is not equivalent to future risk. All other popular metrics of risk (VaR, CVaR, drawdowns, etc.) can be shown to be mathematical transforms of estimated volatility if the distribution of returns is normal, or “normal equivalent” parameters are computed for non-normal distributions. As such, all these metrics suffer from the same insufficiency as simple volatility as a measure of risk. They fail to capture numerous features of the process that invalidate the idea that ex-ante volatility should be an unbiased estimator of realized volatility.
In physics there is a concept known as the Anthropic Principle. Put informally it states: The universe must have evolved the way it did. If had evolved in some other way, we would not exist and be present to observe the alternative universe that might have been. This is important in an investment context because any measure of realized volatility implies survival. In the real world, companies do go bankrupt, bonds do default, and on rare occasions even entire stock markets disappear (Russia 1917, China 1949). To the extent that the probability of such extreme events is non-zero, any estimate of future risk has to be higher than the estimated future return volatility, which is conditional on survival.
While “extinction” events are rare, investors consider them important. Numerous research studies have argued that investors care more about the potential for extreme rare events (“tail risk”) than average risk levels experienced on a day-to-day basis. Such studies include Barro (2005), Gabaix (2009), and Dimson, Marsh, Staunton (2012). It has also been argued that the apparent failure of empirical data to fit the predictions of classic finance models like CAPM is the result of investor fixation on extreme outcomes. Obviously, levered investors such as hedge funds care about the need to avoid being liquidated due to margin calls. It’s like boxing. As a geared fund, getting hit hard enough just once terminates your participation. For long term investors like pension funds and endowments, actuaries routinely use the term “permanent impairment” to describe the situation where a fund has lost so much it may never recover.
To consider the impact of rare extreme events, we will use the example of a “catastrophe bond.” Catastrophe bonds are bonds that are used as a form of reinsurance against natural disasters such as earthquakes. “Cat” bonds pay high yields that are secured by keeping the proceeds in Treasury bonds, while the spread is a paid by an insurer as a premium. They are generally priced assuming a 2% probability of a 90% loss. Our hypothetical security is a long duration “Cat bond” (conditional on no disaster claim) where the annual return will either be 18% (interest rates down) or -2% (interest rates up). The return will be 8% with a standard deviation of 10.05%. With the 2% chance of -90% return included, the return drops to 6.04% with a volatility of about 17%, skew of -3.59% and kurtosis of 18.41. For a long position, the volatility equivalent is 33.13%. For a short position the volatility equivalent is 5.41%. Neither ex-ante value corresponds to the 10.05% that is the only possible ex-post realization. Cornish and Fisher (1937) provides the computation details of volatility equivalents in the presence of higher moments.
Another reason why ex-ante and ex-post estimates of volatility should not correspond is estimation error. To the extent that risk is in the future, future volatility can only be estimated with error. The error can arise in several ways: (1) our estimation process is based on finite samples of data, (2) we can methodological mistakes, and (3) the world can change between the past and the future. Conventional modeling assumes that the parameters of the problem are known with certainty. Let’s consider the situation of a portfolio with beta = 1, market volatility of 20% and zero idiosyncratic risk. In this case, our estimate of future annual volatility is (12*202).5 = 20%.
Now let’s recognize that in the real world we cannot know the value of beta we can only estimate it. Assume our estimate is 1 plus or minus 0.3 (.7 or 1.3). There is a 50% chance that the true beta is around .7 and a 50% chance that the true beta is 1.3. Since the two events are mutually exclusive, they are not independent.
With Estimation Error in Beta Only
Forecast Variance [.7] = ((.7*.7) * (20 * 20)) = 196
Forecast Variance [1.3] = ((1.3*.13) * (20 * 20)) = 676
Forecast variance = .5 * 196 + .5 * 676 = 98 + 338 = 436
Forecast volatility = 436^.5 = 20.88
So, to account for the fact that beta is estimated rather than known, the correct volatility estimate = 20.88. Since we’ve taken the volatility of the market as given at 20% per annum. The proper beta estimate for the portfolio is 20.88/20 = 1.044. In the real world, we don’t know that the future volatility of the market will be 20 either. Let’s assume that this also an estimate and that the estimate is 20%. So now we have 4 possibilities, with two values of beta and two values of the market volatility.
With Estimation Error in Beta and Market Volatility
Forecast Variance [.7,15] = 110.25
Forecast Variance [1.3,15] = 380.25
Forecast Variance [.7,25] = 306.25
Forecast Variance [1.3,25] = 1056.25
Forecast variance = .25 * 100.25 + .25 * 380.25 + .25 * 306.25 + .25 * 1056.25 = 463.1
Forecast volatility = 463.1^.5 = 21.52
The problems associated with unbiased estimates of ex-ante volatility are amplified when we use poorly designed metrics. Many risk metrics such as Value at Risk are mathematically incoherent. They obviously don’t make economic sense in certain cases. A good illustration is one purported risk metric that has been widely marketed. In this measure, you first calculate with 95% confidence the worst rate of return that the investor is likely to experience over the next six months. You then map the result into a scale from 1 (no risk) to 99 (worse than -55% return). While this is an appealing concept in terms of using a numeric scale to describe risk tolerance rather than words like “conservative or aggressive” it has some serious shortcomings.
Consider two investments, A and B. With A I have a 95% chance of getting a return of -10 or better. In the other 5% of events I expect to lose 11%. With B, I have a 95% chance of getting a return of -8 or better. In the other 5% I expect to lose 100%. This measure would say that the B is less risky than A (the 95% confidence boundary is less bad). Even more extreme, think back to our catastrophe bond example. In this case, the proposed metric value is 1 indicating very little risk. There is a 98% chance the bond will not default, so there is a 98% chance my loss will be less severe than -1% over six months. Since 98% is greater than 95% this investment apparently has almost no risk, as opposed to our previous estimate of a 33% annual volatility, or 23.4% per six months. In fairness, it should be noted that incoherence is mitigated when we know a priori that the return distribution is normal, or when we use volatility equivalent measures in presence of higher moments.
To make matters worse, it is routine for risk model providers to “calibrate” their models by observing the relative values of ex-ante volatility forecasts and the realized volatility observations. The parameters of the model are adjusted in hindsight until the ex-ante and ex-post measures agree on average. While this seems sensible at first glance, it amplifies the problem that extreme events are rare. As such, extreme negative events are not present in most observed realization periods. Calibrating models to what did happen rather than what could have happened clearly downward biases the estimation of risk. If we calibrate the model that underlies an incoherent metric, we are effectively “doubling down” on the expectation that nothing extremely bad will happen within the forecast horizon. The potential for extreme events is ignored by the metric, and the data has been adjusted to reflect agreement with observed history that is survivorship biased.
A common approach to addressing these concerns is Bayesian statistics. Bayesian statistics rejects the idea of unbiased estimates of unknown variables. The Bayesian approach looks for an efficient estimate of the unknown, which accounts for the fact that the consequences of making an estimate too high or too low may be radically different. Consider estimating the degree of flood protection for a nuclear power plant. While having too much protection might waste money, underestimating the need for protection could cause massive loss of life. Clearly, the consequences of error are not symmetric. The mathematician and philosopher Blaise Pascal spent many years trying to prove the existence of God. He eventually gave up and roughly concluded: A wise man will believe in God, because the negative consequences of believing that God exists if he does not are minimal. Failing to believe in a God that does exist has substantial negative consequences.
We can understand the implication of this on the arithmetic of investment. If investors are only concerned with wealth change in a single period, then a 50% gain and a 50% loss are equivalently important. However, if investors are concerned with outcomes over multiple periods, then terminal wealth is a convex function of volatility (risk adjusted return is linear in volatility squared). If you lose 50% of your money in one period, you have to make a 100% return in the next period just to get back to your starting wealth despite a 25% average return per period. In the vast majority of circumstances, investors are risk averse as is consistent with both classic utility definition (Bernoulli, 1715) and behavioral finance concepts like prospect theory. As such, the economic consequences of underestimating risk and overestimating risk are not symmetric. Investors should normally prefer to overestimate risk to underestimating risk. Unbiased estimates are not simply desirable.
The fact that not all ex-post observations are equivalent should also be considered. We’ve already established that any comparison of ex-ante versus realized volatility is survivorship biased. Another form of this problem is when we carry out ex-ante versus ex-post comparisons under the assumption that we are indifferent to whether the observation represented a high volatility or low volatility period. For example, a residential smoke alarm signals fire danger. It is obviously more important that the alarm work properly when the house on fire, rather than work properly when the house is not on fire. The worst outcome of one could be loss of life and property. The worst outcome the other would be the inconvenience of a false alarm.
One of the interesting outcomes of the faulty comparison of volatility and risk is that investment professionals are often overcompensated for performance bonuses tied to risk adjusted returns. This often happens when the metric is the Sharpe ratio, or information ratio. By downward biasing the denominator, we upward bias the ratio. Investors are not blind to this issue. Many years ago I had to testify as an expert witness in US Federal Court in a dispute between a family office and their investor clients (i.e. relatives). The investors asserted fraud on the grounds that they had made 65% in 18 months on what were supposedly conservative portfolios. They believed the investments had to have been very risky to have so much upside. The investors cared more about what they thought might have happened than what did happen.
Put simply, volatility and risk are not equivalent concepts. When we equate volatility and risk, it is no more valid than asserting that “apples” and “fruit” are equivalent concepts, whether ex-ante or ex-post. Grammatically this is a false syllogism. The process of judging the efficacy of a risk model based on unbiased estimation of realized volatility is inherently flawed for several reasons, both statistical and economic in nature. In every case, the expected value of future risk is greater than the expected value of future volatility. The practical consequence of this misunderstanding is that asset managers routinely take more risk than they think they are, and are often paid too much for actively pursuing biased tradeoffs between return and risk.
Research Webinar - Valuing Liquidity: Estimating the Price of the Option to do “Something Else”
July 28, 2022 - 11:00 AM EDT
Presented by Dan diBartolomeo
The Northfield/CQF Video Course on Investment Risk is also Now Available. This is a ten-episode educational video series produced by Northfield and the CQF Institute. Hosted by Dan diBartolomeo.
About the Author:
Mr. diBartolomeo is President and founder of Northfield Information Services, Inc. Based in Boston since 1986, Northfield develops quantitative models of financial markets. The firm’s clients include more than one hundred financial institutions in a dozen countries.
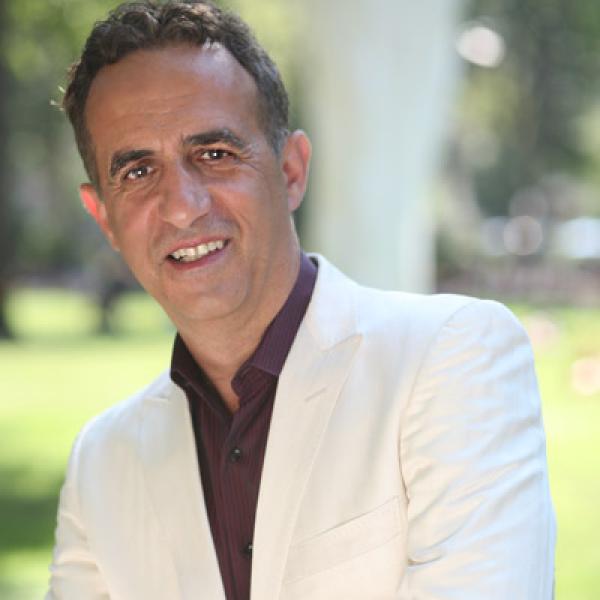
Dan serves on the Board of Directors of the Chicago Quantitative Alliance and is an active member of the Financial Management Association, (“QWAFAFEW”), the Society of Quantitative Analysts. Mr. diBartolomeo is a Director of the American Computer Foundation, a former member of the Board of Directors of The Boston Computer Society, and formerly served on the industry liaison committee of the Department of Statistics and Actuarial Sciences at New Jersey Institute of Technology.
Dan is a Trustee of Woodbury College, Montpelier, VT and continues his several years of service as a judge in the Moscowitz Prize competition, given for excellence in academic research on socially responsible investing. He has published extensively on SRI, including a forthcoming book (with Jarrod Wilcox and Jeffrey Horvitz) on portfolio management for high net-worth individuals.