By Ashby Monk, Executive Director at GPC Stanford University
Sheridan Porter, Co-founder of FEV Analytics
Rajiv Sharma, Research Director at GPC Stanford University
The SEC’s Asset Management Advisory Committee noted in its recent report[1] that retail investors holding private assets in their retirement plans may find that the reputed performance of private equity firms does not translate logically to their account balance. We might be tempted to dismiss this problem as a lack of financial sophistication on the part of retail investors. However, the reality is that the industry’s principal metrics – the IRR and MOIC – are inadequate measures of performance. And, upon their properties rests a broader transparency problem that, ultimately, impedes the efficient allocation of capital. Given the societal and environmental grand challenges we need the capitalist system to now help solve, this lack of transparency has become an urgent problem. And even asset managers have become attune to this issue given plans to expand into the defined contribution (DC) retirement plan market; the allure of the retail investor may just be the carrot they need to join in the march toward transparency.
Although behavioral elements undoubtedly contribute to the industry’s general state of opacity, the problem needs to be brought into sharper focus for it to be meaningfully addressed. In the public markets, continuously observable price informs performance measurement and a robust body of econometric research and technology development. Its absence in the private markets has resulted in a relative paucity of quantitative research and distinctly different performance measures taking root, from which we identify three drivers of opacity:
- Intransitive Metrics
In mathematics, transitivity refers to the logic of relations such that if A > B, and B > C, then A [must be] > C. The IRR has intransitive properties, which drives opacity by making logical comparisons (and rankings) inconclusive, and aggregation disorderly.
- Risk Misalignment
The 2-8-20 profit-share compensation structure creates a risk asymmetry in favor of the GP. It incentivizes risk without specifying risk thresholds, and via numerous mechanisms, permits the GP to accelerate their right to profits while adding weight to the risk-reducing effect of the management fee portion.[2]
- Net-of-Fees Paradigm
In addition to shrouding potential misconduct, net-of-fees reporting builds net-of-fees data sets, which are unable to support rigorous modeling of active management (GP or LP) and other forms of relative performance.
These issues mean that even with full and forthcoming disclosure between a GP and LP, such as with past performance data shared at fundraising, transparency is still thwarted. The lack of transparency in the private markets should therefore be considered structural in origin and fundamentally a measurement problem.
A Framework for Transparency
Organic Finance is a research project at Stanford University’s Global Project Center that examines transparency as a performance driver in investing. It describes transparency as a pathway of observable facts between the asset owner and the value-and-risk-producing base asset. The power to process these facts into actionable intelligence, i.e., measurement, is the domain of data science technologies. The key ingredient of organic finance – and transparency – is therefore data.
We note, however, that in private equity, deficiencies in investment data have led to a vicious data cycle that has ossified the industry’s performance metrics. As indicated in Figure 1, stimulating a virtuous data cycle, that in turn sets the preconditions for scientific measurement, can start with a step as simple as GPs providing reported data in spreadsheet form.
However, a more forward-compatible and audit-friendly solution is a digital information flow where reporting data is machine readable, human readable, dimension-specific, and tethered to accounting standards.[3] Fortunately, this is not as far-fetched as may be anticipated; extensible business reporting language (XBRL) offers a universal financial reporting framework that meets all these requirements and is already in widespread use. Since the change to XBRL is essentially one of reporting format (rather than substance), GP cooperation need not be contingent upon a legal consensus of the fund’s LPs. Thus, a reporting protocol (not a template) is able to fit naturally into existing reporting processes without overreach or being at all prescriptive to GPs.
Data flow describes the movement of data through a ‘security network’ – the machines and software of authorized parties – to compile the targeted ‘pathway of facts’ or transparency between the LP and the base asset (see Figure 2). Critical to the integrity of the network is the concept that data may be added to the flow but not removed from it – like immutable data principles we see in blockchain. In this way, even if an asset management chain is nested multiple times over, the final LP still has transparency. Achieving this is not burdensome to the GP or the network because it does not represent a requirement for new, additional data and it removes the profligate waste of multiple data extraction and reingestion cycles from the network.
To avoid valuation introducing delay and unpredictable bias to the data flow, valuation should be approached as a measured quantity produced by a scalable, systematic process.
In this way the valuation utility can sit in the data flow at any point as a producer of primary intelligence, on top of which unambiguous time-weighted performance measurement can be calculated. Commercially available technologies are a mix of artificial intelligence and data science, which a review of the literature reveals is already in implementation by forward-leaning LPs,[4] and, endorsed by industry groups for their ability to perform critical processes such as daily valuation of alts in 401(k) plans.[5]
There may be some cultural resistance to a valuation intelligence because conventionally, valuation relies on subjectively chosen variables to influence outputs – the proverbial ‘black box’ problem. But a modern approach is different. Data science models learn from data in which subjectivity is implicit, replacing the need for subjectively chosen quantities in core throughput. In nuanced situations (e.g. valuation of pre-revenue companies) where additional adjustment may be desired, it can be applied as a kind of overlay to core model outputs and made explicit and comparable, i.e., transparent. Core model outputs are repeatable, meaning the same inputs render the same output regardless of operator (using the same technology). Testing valuation technology is systematic, automated, and involves millions of data points. It is in stark contrast to the coarse sampling examinations of conventional valuations, if testing is done at all. The scientific process enshrines, for the first time, evidence-based practice, scalable testing, and the property of repeatability around a central component of investor intelligence: valuation.
The final component of transparency is performance comparability, necessitating the measurement of relative returns. To ensure integrity of the comparison, the construction of the benchmark should be – like valuation – a measured output and produced by a scalable, systematic process. In line with our definition of transparency, the measurement should target the holdings level and ‘roll up’ into aggregations such as the fund or as otherwise defined by the operator (as shown in Figure 3). A review of the literature shows the successful application of non-market-cap-weighted indexing technology to the PME task.[6]
The authors show the indexing technology maintaining a highly specific and stable idiosyncratic/systemic boundary through cashflows and extended holding periods to resolve well-known issues with the conventional PME. A next-generation PME utility in the data flow produces novel intelligence needed to innovate compensation structures, alignment of interests, liquidity algorithms, and risk management within the portfolio.
Transformative Potential of Transparency
Transparency is sometimes presented as a zero-sum game, focused almost exclusively on fees, in which LPs win and GPs lose. But, in solving the measurement problem, a structural limitation is removed for all industry participants. For reluctant GPs, PE is already facing challenges to its performance claims and its ability to align with societal needs. It is unlikely that the industry can continue scaling up without addressing these concerns. Rather than bear unknown distortions from an imposed regulatory constraint, transparency from scientific measurement puts into play new opportunities for industry expansion, financial innovation, operational efficiency, and efficient allocation of capital.
For LPs, measurement and benchmarking technologies targeting the holdings level provide a new potential source of edge and efficiency in important LP functions, such as manager evaluation, liquidity management, manager seeding, risk management, benchmarking, and operational innovation (such as removing reporting lag). For GPs and asset managers, the same measurement technologies can be applied differently (see Figure 4 for example), including rigorous substantiation of their value-add and sources of alpha, meeting demand for more frequent valuation and expanded service levels, tools to optimize capital structure and growth, and the means to proactively bring differentiated risk-aligned compensation structures to market. We provide many specific examples of transparency-driven opportunities for differentiation, indexation, cost reduction, and innovation – for all industry participants – in An Economic Case for Transparency in Private Equity: Data Science, Interest Alignment and Organic Finance, available for download from SSRN.[7]
To the industry, however, the most pronounced contribution of transparency is to the understanding and management of risk, including business and operational risk that limits the industry. As a niche example, retirement plan sponsors concerned about legal exposures are seeking transparency (and its tools) to lower operational risks related to valuation, liquidity, and fees. In this case, lower operational risks translate to lower legal risk.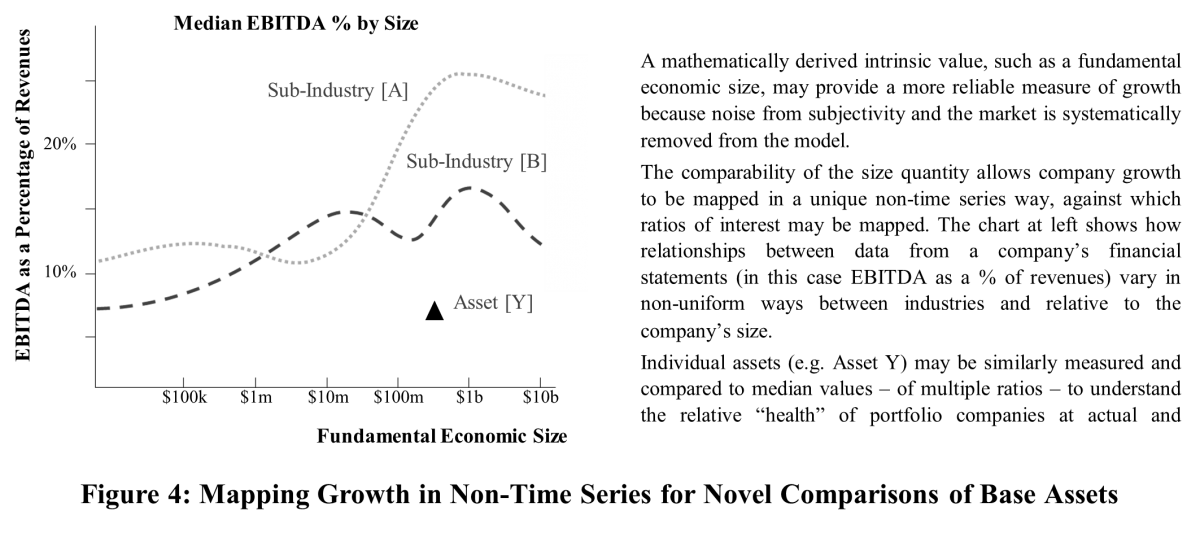
More generally, transparency means that risk frameworks can be enhanced to include monitoring of leverage levels and liquidity ‘contagion’ pathways in the portfolio. In testimony before the U.S. House of Representatives in 2009, Dr. Richard Bookstaber, noted author of financial risk management, explained the liquidity crisis cycle and its modes of contagion as observed in the 2008 financial crisis.
There is room, he argued, for a regulatory body to exercise a hilltop view for the purpose of anticipating crises by measuring asset-level data (like leverage and loan volumes) across multiple large firms. Albeit in a much smaller universe, the same theoretical oversight could be developed by LPs using asset data, holdings data, and securities data from every GP in their own portfolio. By measuring their own universe, LPs could provide a form of collective governance over the market, which in theory, would provide a natural check against risk accumulation within the system.
Conclusion
In research published in 2020, the University of Oxford’s private equity expert, Phalippou, described at least ten years of the asset management industry taking substantially all excess returns, leaving LPs with a net-of-fees return equal to public market returns. However, during the same period, the size and scope of the private capital markets grew every year precisely because of a convincing economic case. The simultaneous existence of these two opposing viewpoints, both informed by accepted quantitative analysis, exemplifies the pressing need for performance clarity. As we have shown, the path forward need not be contingent upon regulation, LP overreach, or GP beneficence. Instead, all parties can play a role in seeding a virtuous data cycle, and all parties can benefit from the transparency it provides.
Footnotes:
[1] U.S. Securities and Exchange Commission, Asset Management Advisory Committee, Final Report and Recommendations for Private Equity, Sep. 27, 2021.
[2] GPs may waive their management fee in exchange for LPs accepting the GP investment (in the fund) as ‘in-kind’. However, this creates a tax arbitrage because (at the time of writing) management fees received by the GP are deemed ordinary income, and taxed as such. If management fees are instead deemed invested capital, proceeds may be regarded as capital gains and taxed accordingly [at the more attractive rate]. This reduces the real capital committed by the GP and further lowers their risk. Consider also that in-kind arrangements mean GPs do not have to meet urgent and unpredictable capital calls, reducing their liquidity-related costs.
[3] Hoffman, C. (2020). Mastering XBRL-based Digital Financial Reporting. Working ed. [online] Available at: www.xbrlsite.com/mastering/contents.html
[4] Guimarães, A., Monk, A., and Porter, S., (2018). Improving Investment Operations through Data Science: A Case Study of Innovation in Valuation. The Journal of Portfolio Management, 45(1), pp. 125 – 140.
[5] DCALTA (2021). Daily Valuation of Alternative Assets in DC Plans. [online] Available at: https://d9ff0f78-cad3-413e-8fb0-dc6302cc7851.filesusr.com/ugd/1bf9e3_10124ed71c354aa2affcc23f5018f42b.pdf
[6] Porter, S. and Porter, S. (2019). Introducing Objective Benchmark-Based Attribution in Private Equity. The Journal of Financial Data Science, 1 (1); 130-140.
[7] Monk, A., Porter, S., and Sharma, R. (2021). An Economic Case for Transparency in Private Equity: Data Science, Interest Alignment and Organic Finance. Working ed. [online] Available at: https://papers.ssrn.com/sol3/papers.cfm?abstract_id=3931906
About the Authors:
Dr. Ashby Monk leads Addepar’s research team, which develops insights to fuel the creation of content, products and services that offer value to RIAs, family offices, and institutional investors. Ashby joined Addepar when it acquired Real Capital Innovation (RCI), the company he co-founded, in 2020.

Ashby also co-founded Long Game and FutureProof Technologies, startups in the financial services and tech space. Ashby continues to serve as the Executive and Research Director of the Stanford Global Projects Center. He was named one of the most influential academics in the institutional investing world by CIO Magazine. Ashby earned a doctorate in economic geography from Oxford University, a master's in international economics from Pantheon-Sorbonne University, and a bachelor's in economics from Princeton University.
Sheridan Porter is co-founder of FEV Analytics, a Seattle based fintech firm pioneering valuation science for the private capital markets.
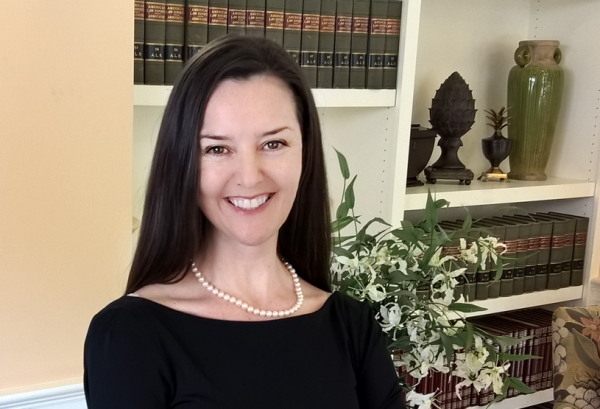
Within the industry she is a prolific technology evangelist, helping stakeholders gain clarity on structural impediments to technology adoption, and how technology can be deployed to achieve transparency and greater operational efficiency. She has collaborated on and authored numerous technical and theoretical papers on these subjects and is leading industry efforts to improve its data operationalization.
Sheridan previously served in C-level roles for US and Indian information technology companies, and as a clinical trials manager in the Australian healthcare system. She is a former member of the Australian synchronized swimming team, a proud mother of two youngsters, and holds a Master of Business Administration from Melbourne Business School.
Dr. Rajiv Sharma is a Research Director at Stanford University’s Global Projects Center and Product Manager at Addepar. He is also a special advisor to the US Department of Treasury Office of Technical Assistance Government Debt and Infrastructure Finance team.

Dr. Sharma is the author of multiple peer-reviewed publications on innovation in institutional investment including the book, Reframing Finance. He has worked in Venture Capital and PE/Infrastructure Advisory, consulted with a number of global institutional investors and governments and worked with the World Bank, United Nations and OECD. He has a PhD from Oxford University and Bachelor of Engineering and Commerce (hons) from the University of Auckland.